Plotting a DataFrame
This is the last lesson of the course and shows you how you can plot your final DataFrame using vincent
.
Resources
- More about vincent: official documentation
- More about data visualization: Interactive Data Visualization in Python With Bokeh
Congratulations, you made it to the end of the course! What’s your #1 takeaway or favorite thing you learned? How are you going to put your newfound skills to use? Leave a comment in the discussion section and let us know.
00:01
We’re going to create a stacked graph, which is going to be represented by vincent.StackedBar()
, which takes a DataFrame
, which in our case is the field_goal_per_team
.
00:11 We’re then going to create a legend for this
00:16
particular one, which is going to be a title
, and it’s going to be "Field Goals"
.
00:27 Once we have that together, we’re then going to scale our x-axis so it’s spaced out cleanly. I only know this because I’ve done this before. It’s usually something you have to see with your particular dataset to figure out what looks nice, so this is mostly trial and error, but I know that works well for the particular
00:50
set that I’m working with. And then we’re going to simply go stacked.display()
.
00:58 So as you can see, we have a breakdown of each team, how Kevin Durant performed against each team in terms of field goals attempted and field goals. So as you can see, the field goals he scores are much less than the ones he attempted.
01:10 So he attempted about 40 and he scored about 20 of those against Atlanta. As you could see from before, that is about right. Where are you? He attempted 40, he scored about 20, which is quite awesome.
01:25 So you can see he doesn’t perform very well against Milwaukee. That could have been for various reasons, it could have been because he was injured or did not get that much playtime.
01:33 If you were to have another dataset of whether his injuries had occurred, you can easily show games where he’s injured or he was hurt the previous game or sick—you could see how his performance varies against those days.
01:46 And this is very much more consumable than anything else, and you can share this with friends and whatnot. This concludes our more in-depth talk on DataFrames.
myPyTeck on March 12, 2020
He played four matches against DAL vs one against MIL. Please use what you have shown for averages and correct the plot.
myPyTeck on March 12, 2020
I cut the DataFrame to columns I will need for the plot only
group_by_opp = data.groupby('Opp')[['FGA', 'FG']]
I divided FGA and FG sums by number of matches played
field_goals_per_team = group_by_opp.sum()/group_by_opp.count()
And finally I’ve got much more informative plot
stacked = vincent.StackedBar(field_goals_per_team)
stacked.legend(title='Field Goals')
stacked.scales['x'].padding = 0.1
stacked.display()
zbigniewsztobryn on April 26, 2020
Great tutorial - thanks!
sroux53 on May 14, 2020
Excellent!
khurram703 on June 26, 2020
I m trying to run the below code in idle and it is not showing me the graphs in idle. Is idle not compatible with vincent? What i have to do make it working?
vincent 0.4.4 is already installed in my pc
stacked = vincent.StackedBar(field_goal_per_team)
stacked.legend(title='Field Goals')
stacked.scales['x'].padding = 0.1
stacked.display()
Output in idle:
<IPython.core.display.HTML object>
sunflower761 on Sept. 5, 2020
Thank you for the informative lesson
Satish on May 12, 2021
This was very different from the usual quality of video courses at RealPython. Would be better if this course provides links to the first 2 introductory Pandas tutorials ( in the learning path ‘Pandas for Data Science’) so that it would be helpful for others
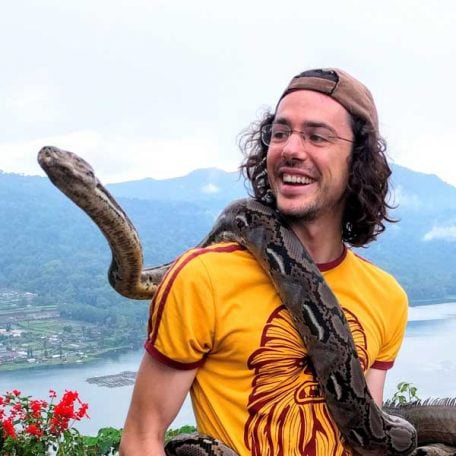
Martin Breuss RP Team on May 12, 2021
hi @Satish! I’m not entirely sure what you mean 🤔 Did you not find the course helpful? Happy to hear your thoughts and suggestions.
In any case, here’s the link to the Pandas Learning Path in case this might be helpful for someone.
macro84 on Nov. 7, 2021
Best to provide a link to the kevin.csv used in the tutorial. Perhaps the Basketball website changed the format? Good to learn vincent. Good to learn MAP and GROUPBY but it would have been more helpful if I had been able to get the kevin.csv in the right data format. All the dtypes were “object”. I need to learn some dataframe basics to learn to fix these issues.. Here are some of the outputs… 1) time data ‘Inactive’ does not match format ‘%M:%S’ 2) unsupported operand type(s) for /: ‘str’ and ‘str’
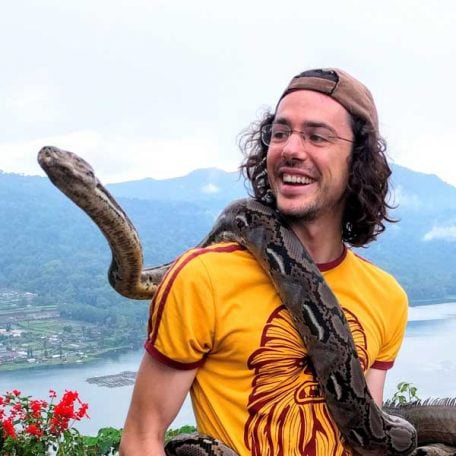
Martin Breuss RP Team on Nov. 8, 2021
Hi @hlaret this course is a little outdated and the vincent project isn’t maintained anymore might not be the most popular visualization library to learn at this point.
If you’re looking for a very similar project that is based on Vega as well, you might want to check out Altair, which is currently still actively maintained.
Alternatively, if you just want to get started with plotting in Python, you might want to take a look at one of these two courses instead:
- Plot with Pandas: Python Data Visualization Basics or
- Interactive Data Visualization in Python with Bokeh
Hope this helps! Projects come up and get discontinued all the time… Sometimes this can be frustrating, but you’ll see that learning one library will help you better understand other libraries, even if the first one you learned won’t make it across the harsh threshold of time :P
macro84 on Nov. 9, 2021
Thanks appreciate the feedback! Real Python is great. Loving it so far.
Become a Member to join the conversation.
pshekhar2707 on March 5, 2020
good to learn about vincent visualisation pkg