For more information on what you can do with grouping and aggregating, check out pandas GroupBy: Your Guide to Grouping Data in Python.
Grouping and Aggregating Your Data
00:00
Take a look at the city_revenues
Series
again. You can get the total of the values in this Series
by calling the .sum()
method or the maximum value in the Series
with the .max()
method.
00:15
And there are additional aggregation methods, including .min()
, which gets the minimum value, and .mean()
, which gets the average value.
00:26
A column in a DataFrame
is a Series
, so you can call those same methods on a column in a DataFrame
like this. Now take a look at the 'fran_id'
(franchise ID) column in the nba
DataFrame
.
00:41
There are only a few unique values in this column. You can group the rows in the DataFrame
by the value of the 'fran_id'
column. However, the return value isn’t very useful directly.
00:57
Instead, you can call the aggregation methods and they will be applied to each group. Notice the sort
keyword to the .groupby()
method.
01:08
If you have a large DataFrame
and the order is irrelevant, sorting can cause performance issues. Setting sort
to False
can prevent some of these problems.
01:21 You can also group by and aggregate multiple columns. This would group rows first by year, and then it will create subgroups inside of each year for games won and games lost.
01:35 And you can count the total number of games won and lost for each year.
01:42
How many games did the Golden State Warriors win or lose in the year 2015? First, query the nba
DataFrame
as you learned in the previous lesson.
01:55
Filter the 'fran_id'
for 'Warriors'
and the 'year_id'
for 2015
. Then group by the 'game_result'
and count the games lost and won.
02:08
Was their record better in the playoffs? By adding the 'is_playoffs'
column to the .groupby()
, the games will be first grouped into playoff and regular season and then by wins and losses. Notice that when grouping a single column, use just the string name, but when grouping more than one column, use a list of names. There’s much more you can do with grouping and aggregating. Check out this post on Real Python for more.
02:37 In the next lesson, you’ll learn more about DataFrames by manipulating the columns.
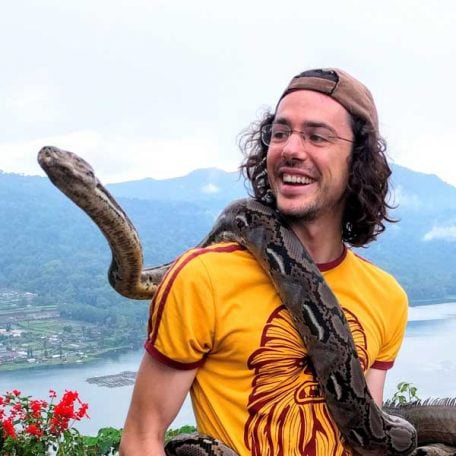
Martin Breuss RP Team on Oct. 21, 2021
Hi @Kim you’ve done everything correctly and in fact discovered a small typo in the lesson recording. The method on the pd.Series
object should also be .sum()
(without the second m
).
That second letter must have accidentally sneaked in there right after Douglas executed the code cell, otherwise he’d also have bumped into the same error as you did. I’ll see if we can get that fixed in the video. Thanks for the heads-up!
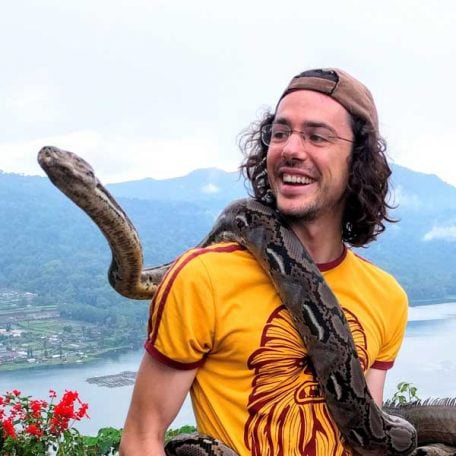
Martin Breuss RP Team on Oct. 22, 2021
Thanks again @Kim for finding this, we got it fixed in the lesson video, so now it’s showing the correct method name, .sum()
🙂
Kim on Oct. 28, 2021
Thank you very much for checking into it!
Cindy on July 19, 2022
Hi Martin, in terms of counting game results, I am wondering what is the reason to add 'game_id'
for the code: year_results['game_id'].count
? Can we just write: year_results.count
? Thank you.
Become a Member to join the conversation.
Kim on Oct. 21, 2021
Hi, I am going through the “Grouping and Aggregating Your Data” lesson in the Explore Your Dataset with Pandas Course. In following the lesson, I am reproducing the code in the course into my own notebook.
When I tried to use the
I ended up with an AttributeError: ‘Series’ object has no attribute ‘summ’
I am not far enough in my understanding on how to correct this. Any hints? As far as I know I have not done anything differently in my coding from what the instructor has demonstrated.
Thank you!