Working With SQL
00:00 Working with SQL files. pandas IO Tools can also read and write databases. In this next example, you’ll write your data to a database, but to get started, you’ll need to install the SQLAlchemy package, as seen onscreen now.
00:20
In addition, you’ll also need a database driver. Fortunately, Python has a built-in driver for SQLite databases, so that’s what we’ll be using. Once you have SQLAlchemy installed, import create_engine()
and create a database engine as seen onscreen.
00:49
Now that you have all that set up, the next step is to create a DataFrame
object as seen onscreen.
01:22
.astype()
is a convenient method you can use to set multiple data types all at once using a dictionary as seen onscreen. Now the DataFrame can be saved to a database using the .to_sql()
method as seen onscreen.
01:46
The parameter con
is used to specify the database connection or engine that you want to use. The optional parameter index_label
specifies how to call the database column with the row labels.
01:57
You’ll often see it take on the value 'ID'
. This code had produced the file data.db
, which can be read with an SQL file viewer.
02:08
The first column contains the row labels. The other columns correspond to the columns of the DataFrame. To omit writing row labels into the database, pass index=False
to the .to_sql()
method, and this will lead to the database seen onscreen now. There are a few more optional parameters, for example, you can use schema
to specify the database schema and dtype
to determine the types of the database columns. You can also use if_exists
, which says what to do if a database with the same name and path already exists.
02:46
if_exists='fail'
raises a ValueError
and is the default. if_exists='replace'
would drop the table and insert new values.
02:56
if_exists='append'
inserts new values into the table. You can load the data from the database using read_sql()
.
03:15
The parameter index_col
specifies the name of the column with the row labels. Note that this inserts an extra row after the header that starts with ID
.
03:26 You can fix this behavior with the following line of code.
03:39
Now you have the same DataFrame
object has before. Note that the content for Russia is now None
instead of nan
. If you want to fill in the missing values with nan
, then you can use .fillna()
as seen onscreen.
04:05
.fill_na()
replaces all missing values with whatever you pass to value
. Here, you passed float('nan')
, which says to fill all missing values with nan
.
04:16
Also note that you didn’t have to pass parse_dates=['IND_DAY']
to read_sql()
. That’s because the database was able to detect that the last column contains dates. However, you could pass parse_dates
if you’d like, but you’d still get the same results.
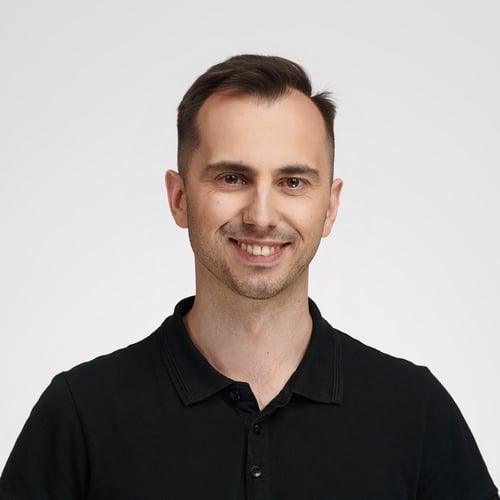
Bartosz Zaczyński RP Team on Sept. 24, 2021
@KatMac I think you want to transpose the data frame after having converted column types. Otherwise, the columns will have names corresponding to the consecutive numbers 0, 1, 2, and so on.
Try this instead:
db_df = pd.DataFrame(data=data).astype(dtype=db_types).T
KatMac on Sept. 24, 2021
Thank you Bartosz…
In my code, I have updated the db_df line as you suggested. The previous error has disappeared. When I run the code now the following displays (partial display to save space)
0 object
1 object
2 object
3 object
4 object
....
19 object
dtype: object
Cindy on July 4, 2022
Hi Bartosz, thanks for the lecture. Could you please explain what does ‘echo=false’ mean? Thanks a lot.
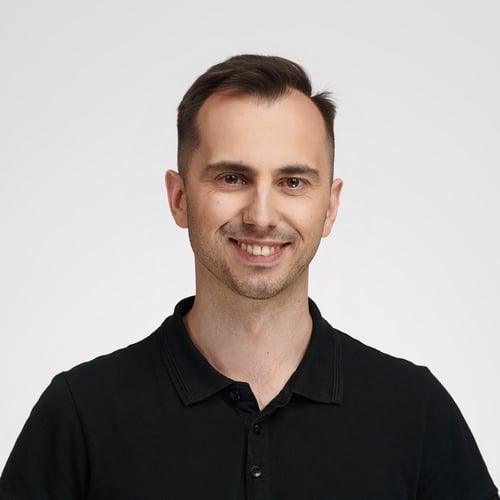
Bartosz Zaczyński RP Team on July 4, 2022
@Cindy The lecture and the whole course were made by Darren, while I only commented on it 😊
The best place to learn about the parameters of a function is the official documentation. In this case, you want to take a look at SQLAlchemy’s documentation.
You’ll find the following description there:
if True, the Engine will log all statements as well as a repr() of their parameter lists to the default log handler, which defaults to sys.stdout for output. If set to the string “debug”, result rows will be printed to the standard output as well. The echo attribute of Engine can be modified at any time to turn logging on and off; direct control of logging is also available using the standard Python logging module. (Source)
Coxy on Nov. 1, 2023
Hi, I am learning Python in VScode - new to programming. Could anyone recommend a viewer to look at the data.db
file that has been created with the code in this course please? Thank you
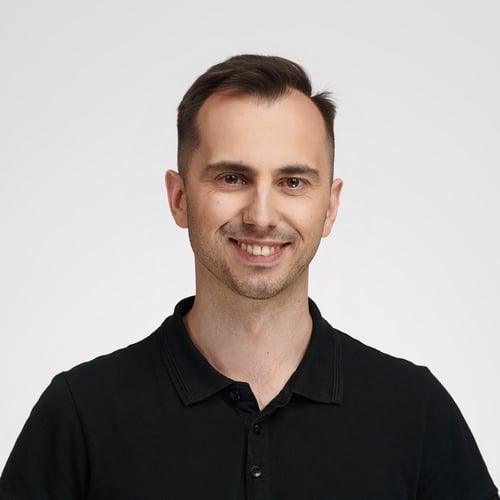
Bartosz Zaczyński RP Team on Nov. 2, 2023
@Coxy It’s a SQLite database, which means that you can use the sqlite3
command in your terminal or install an application with a graphical user interface, such as the dedicated DB Browser for SQLite. Alternatively, you can install a universal SQL viewer like SQuirreL SQL Client or find a Visual Studio Code extension like SQLTools to browse the data without leaving your IDE.
Become a Member to join the conversation.
KatMac on Sept. 23, 2021
Hi I have typed in the following code.....
and am receiving the following error:
Question.
What have I done wrong? and Why did we not assign the COUNTRY type to text in db_types?